This article has been reviewed according to Science X's editorial process and policies. Editors have highlighted the following attributes while ensuring the content's credibility:
fact-checked
peer-reviewed publication
trusted source
proofread
Machine learning enables viability of vertical-axis wind turbines
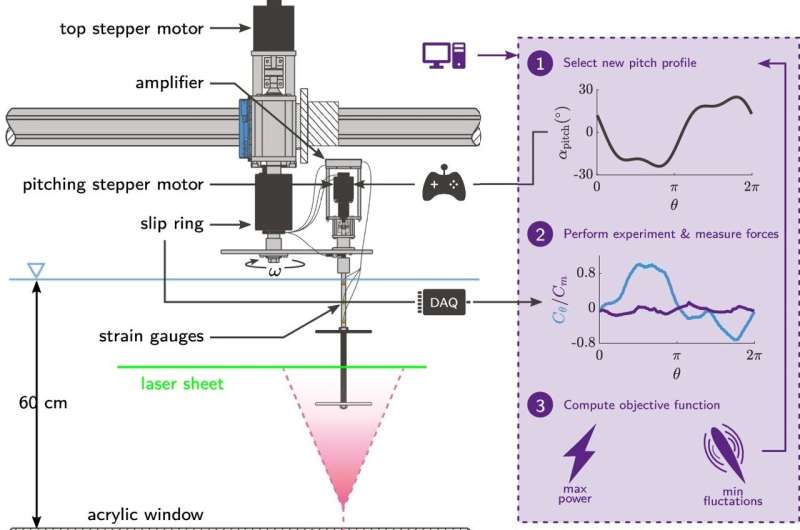
If you imagine an industrial wind turbine, you likely picture the windmill design, technically known as a horizontal-axis wind turbine (HAWT). But the very first wind turbines, which were developed in the Middle East around the 8th century for grinding grain, were vertical-axis wind turbines (VAWT), meaning they spun perpendicular to the wind, rather than parallel.
Due to their slower rotation speed, VAWTs are less noisy than HAWTs and achieve greater wind energy density, meaning they need less space for the same output both on- and off-shore. The blades are also more wildlife-friendly: because they rotate laterally, rather than slicing down from above, they are easier for birds to avoid.
With these advantages, why are VAWTs largely absent from today's wind energy market? As Sébastien Le Fouest, a researcher in the School of Engineering Unsteady Flow Diagnostics Lab ((UNFOLD) explains, it comes down to an engineering problem—air flow control—that he believes can be solved with a combination of sensor technology and machine learning.
In a paper published in Nature Communications, Le Fouest and UNFOLD head Karen Mulleners describe two optimal pitch profiles for VAWT blades, which achieve a 200% increase in turbine efficiency and a 77% reduction in structure-threatening vibrations.
"Our study represents, to the best of our knowledge, the first experimental application of a genetic learning algorithm to determine the best pitch for a VAWT blade," Le Fouest says.
Turning an Achilles' heel into an advantage
Le Fouest explains that while Europe's installed wind energy capacity is growing by 19 gigawatts per year, this figure needs to be closer to 30 GW to meet the UN's 2050 objectives for carbon emissions.
"The barriers to achieving this are not financial, but social and legislative—there is very low public acceptance of wind turbines because of their size and noisiness," he says.
Despite their advantages in this regard, VAWTs suffer from a serious drawback: they only function well with moderate, continuous air flow. The vertical axis of rotation means that the blades are constantly changing orientation with respect to the wind.
A strong gust increases the angle between air flow and blade, forming a vortex in a phenomenon called dynamic stall. These vortices create transient structural loads that the blades cannot withstand.
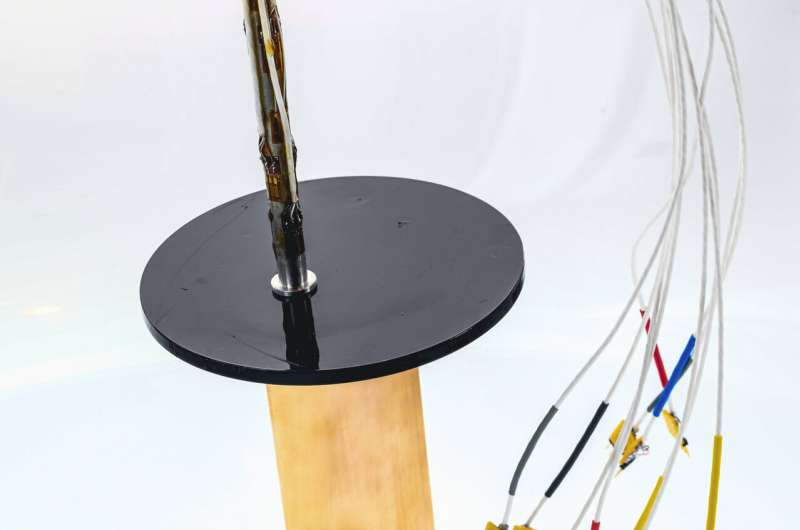
To tackle this lack of resistance to gusts, the researchers mounted sensors onto an actuating blade shaft to measure the air forces acting on it. By pitching the blade back and forth at different angles, speeds, and amplitudes, they generated series of "pitch profiles." Then, they used a computer to run a genetic algorithm, which performed over 3,500 experimental iterations. Like an evolutionary process, the algorithm selected for the most efficient and robust pitch profiles, and recombined their traits to generate new and improved "offspring."
This approach allowed the researchers not only to identify two pitch profile series that contribute to significantly enhanced turbine efficiency and robustness, but also to turn the biggest weakness of VAWTs into a strength.
"Dynamic stall—the same phenomenon that destroys wind turbines—at a smaller scale can actually propel the blade forward. Here, we really use dynamic stall to our advantage by redirecting the blade pitch forward to produce power," Le Fouest explains.
"Most wind turbines angle the force generated by the blades upwards, which does not help the rotation. Changing that angle not only forms a smaller vortex—it simultaneously pushes it away at precisely the right time, which results in a second region of power production downwind."
The paper represents Le Fouest's Ph.D. work in the UNFOLD lab. Now, he plans to build a proof-of-concept VAWT. The goal is to install it outdoors, so that it can be tested as it responds in real time to real-world conditions.
"We hope this air flow control method can bring efficient and reliable VAWT technology to maturity so that it can finally be made commercially available," Le Fouest says.
More information: Sébastien Le Fouest et al, Optimal blade pitch control for enhanced vertical-axis wind turbine performance, Nature Communications (2024). DOI: 10.1038/s41467-024-46988-0