This article has been reviewed according to Science X's editorial process and policies. Editors have highlighted the following attributes while ensuring the content's credibility:
fact-checked
proofread
Synergizing sub-symbolic and symbolic AI: Pioneering approach to safe, verifiable humanoid walking
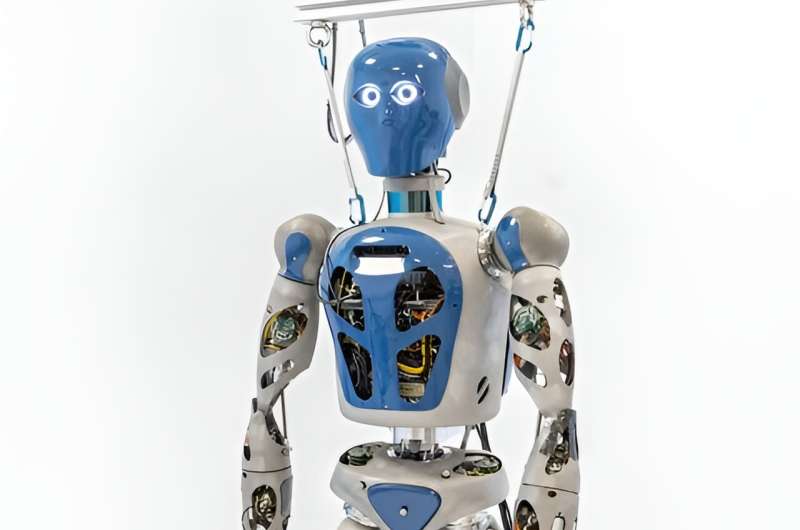
As sub-symbolic AI, like deep learning, continues to advance, its limitations in safety and reliability are becoming more apparent. Verification and stability are crucial in safety-critical domains such as humanoid robotics, which is rapidly evolving into a versatile tool for various applications. However, proving the correctness of AI-based self-learning algorithms is challenging due to their uncertain inferences and opaque decision-making processes.
The German Research Center for Artificial Intelligence (DFKI) in Bremen has developed innovative control methods for complex systems, combining the advantages of fast self-learning and reliable verification via symbolic models. With this hybrid AI approach, the project offers a ground-breaking solution to the challenges of humanoid robotics.
Innovative hybrid AI approach to learn and verify complex robotic behavior
In the VeryHuman project, DFKI researchers addressed these challenges by integrating symbolic and sub-symbolic AI approaches. Specifically, they used symbolic specifications in reinforcement learning, where a system is rewarded for producing results that are mathematically verifiable.
Combining sub-symbolic, self-learning algorithms with those based on mathematical rules and abstractions in a single system has proven difficult. Machine learning decisions are not based on symbolic calculations and cannot be explained by logical rules.
Therefore, DFKI combined the expertise of its two Bremen-based research departments: Robotics Innovation Center, led by Prof. Dr. Frank Kirchner, and Cyber-Physical Systems, led by Prof. Dr. Rolf Drechsler. The goal was to develop an AI-based control system capable of achieving human-like capabilities, particularly in demonstrating safe and stable dynamic walking and other complex movements in humanoid robots.
Deriving rewards for reinforcement learning from symbolic descriptions
By using symbolic specifications in reinforcement learning, such as simple language to describe the robot's behavior, the project team created abstract kinematic models from the system that can be symbolically validated.
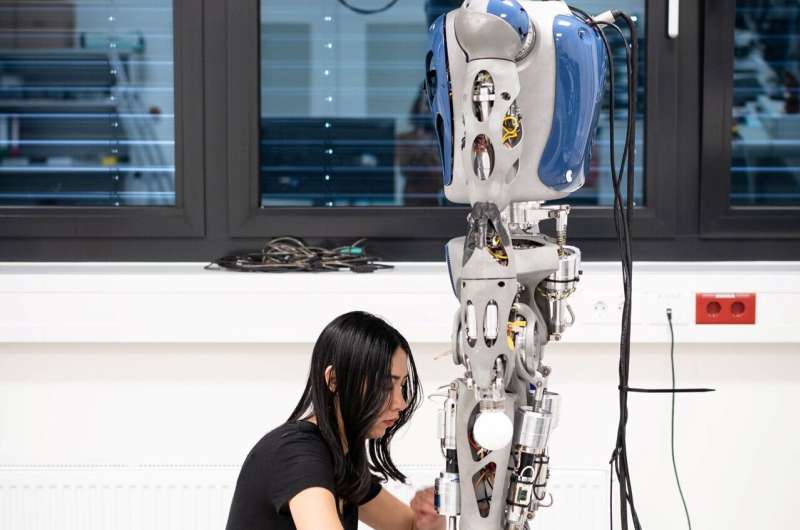
These abstractions allow the definition of reward functions for reinforcement learning and the robot to mathematically verify its decisions based on the models. Thus, the reliability of the system's decisions is improved, ensuring stable and predictable movements, and reducing the risk of misbehavior or unexpected actions.
Additionally, the intended behavior of the robot was modeled as a hybrid automaton, a mathematical model that describes both continuous and discrete behavior. This reduces the system's state space, allowing for more efficient reinforcement learning.
Fast dynamic walking with DFKI's RH5 humanoid robot
Furthermore, the project successfully achieved dynamic walking on DFKI's RH5 humanoid robot by combining the zero-moment point method (the point on a robot's support area where the resulting ground force does not create a tipping moment) with the whole-body control approach in a tailored manner suitable for achieving high performance in position-controlled robots.
This enabled stable and robust dynamic walking at varying speeds and step lengths, effectively pushing the limits of the system in terms of both speed and range of motion.
To the researchers' knowledge, this is the first time a humanoid robot has dynamically walked up to 0.43 m/s. Excluding systems with active toe joints, RH5 is among the fastest humanoids of similar size and actuation modalities. To continuously improve RH5's behavior, the researchers also used simulation and optimal control algorithms based on the symbolic model.
Improved efficiency and safety for AI applications in high-risk areas
Since precise modeling and optimization of motion sequences enhance both the safety and efficiency of robots, the hybrid AI approach developed in VeryHuman can serve as a blueprint for generating reward functions from symbolic AI and reasoning. This is particularly relevant for real-world applications where the safety of robots and their environment is paramount.