CXL-based memory disaggregation technology opens up a new direction for big data solution frameworks
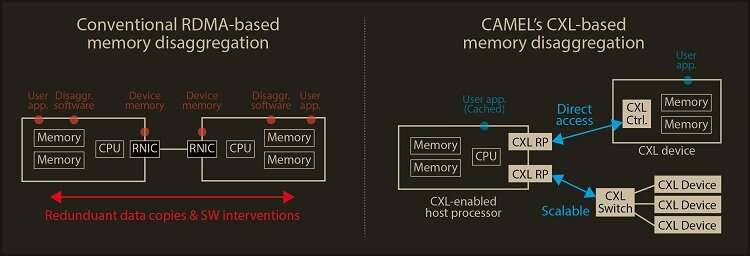
A team from the Computer Architecture and Memory Systems Laboratory (CAMEL) at KAIST presented a new compute express link (CXL) solution whose directly accessible, and high-performance memory disaggregation opens new directions for big data memory processing. Professor Myoungsoo Jung said the team's technology significantly improves performance compared to existing remote direct memory access (RDMA)-based memory disaggregation.
CXL is a peripheral component interconnect-express (PCIe)-based new dynamic multi-protocol made for efficiently utilizing memory devices and accelerators. Many enterprise data centers and memory vendors are paying attention to it as the next-generation multi-protocol for the era of big data.
Emerging big data applications such as machine learning, graph analytics, and in-memory databases require large memory capacities. However, scaling out the memory capacity via a prior memory interface like double data rate (DDR) is limited by the number of the central processing units (CPUs) and memory controllers. Therefore, memory disaggregation, which allows connecting a host to another host's memory or memory nodes, has appeared.
RDMA is a way that a host can directly access another host's memory via InfiniBand, the commonly used network protocol in data centers. Nowadays, most existing memory disaggregation technologies employ RDMA to get a large memory capacity. As a result, a host can share another host's memory by transferring the data between local and remote memory.
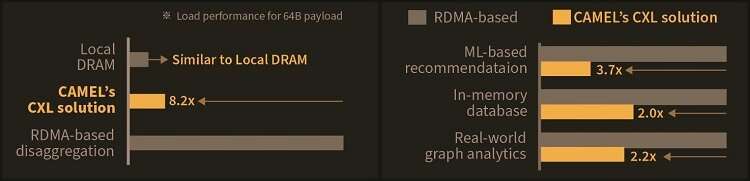
Although RDMA-based memory disaggregation provides a large memory capacity to a host, two critical problems exist. First, scaling out the memory still needs an extra CPU to be added. Since passive memory such as dynamic random-access memory (DRAM), cannot operate by itself, it should be controlled by the CPU. Second, redundant data copies and software fabric interventions for RDMA-based memory disaggregation cause longer access latency. For example, remote memory access latency in RDMA-based memory disaggregation is multiple orders of magnitude longer than local memory access.
To address these issues, Professor Jung's team developed the CXL-based memory disaggregation framework, including CXL-enabled customized CPUs, CXL devices, CXL switches, and CXL-aware operating system modules. The team's CXL device is a pure passive and directly accessible memory node that contains multiple DRAM dual inline memory modules (DIMMs) and a CXL memory controller. Since the CXL memory controller supports the memory in the CXL device, a host can utilize the memory node without processor or software intervention. The team's CXL switch enables scaling out a host's memory capacity by hierarchically connecting multiple CXL devices to the CXL switch allowing more than hundreds of devices. Atop the switches and devices, the team's CXL-enabled operating system removes redundant data copy and protocol conversion exhibited by conventional RDMA, which can significantly decrease access latency to the memory nodes.
In a test comparing loading 64B (cacheline) data from memory pooling devices, CXL-based memory disaggregation showed 8.2 times higher data load performance than RDMA-based memory disaggregation and even similar performance to local DRAM memory. In the team's evaluations for a big data benchmark such as a machine learning-based test, CXL-based memory disaggregation technology also showed a maximum of 3.7 times higher performance than prior RDMA-based memory disaggregation technologies.
"Escaping from the conventional RDMA-based memory disaggregation, our CXL-based memory disaggregation framework can provide high scalability and performance for diverse datacenters and cloud service infrastructures," said Professor Jung. He went on to stress, "Our CXL-based memory disaggregation research will bring about a new paradigm for memory solutions that will lead the era of big data."