January 31, 2023 feature
This article has been reviewed according to Science X's editorial process and policies. Editors have highlighted the following attributes while ensuring the content's credibility:
fact-checked
peer-reviewed publication
trusted source
proofread
A framework that could improve the social intelligence of home assistants
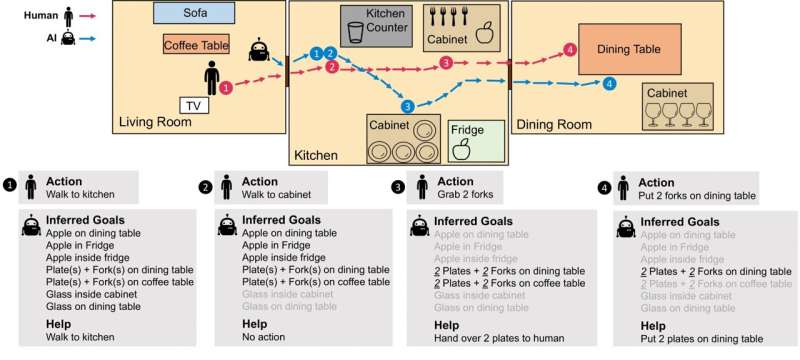
Existing artificial intelligence agents and robots only help humans when they are explicitly instructed to do so. In other words, they do not intuitively determine how they could be of assistance at a given moment, but rather wait for humans to tell them what they need help with.
Researchers at Massachusetts Institute of Technology (MIT) recently developed NOPA (neurally guided online probabilistic assistance), a framework that could allow artificial agents to autonomously determine how to best assist human users at different times. This framework, introduced in a paper pre-published on arXiv and set to be presented at ICRA 2023, could enable the development of robots and home assistants that are more responsive and socially intelligent.
"We were interested in studying agents that could help humans do tasks in a simulated home environment, so that eventually these can be robots helping people in their homes," Xavier Puig, one of the researchers who carried out the study, told Tech Xplore. "To achieve this, one of the big questions is how to specify to these agents which task we would like them to help us with. One option is to specify this task via a language description or a demonstration, but this takes extra work from the human user."
The overreaching goal of the recent work by Puig and his colleagues was to build AI-powered agents that can simultaneously infer what task a human user is trying to tackle and appropriately assist them. They refer to this problem as "online watch-and-help."
Reliably solving this problem can be difficult. The main reason for this is that if a robot starts helping a human too soon, it might fail to recognize what the human is trying to achieve overall, and its contribution to the task might thus be counterproductive.
"For instance, if a human user is in the kitchen, the robot may try to help them store dishes in the cabinet, while the human wants to set up the table," Puig explained. "However, if the agent waits too long to understand what the human's intentions are, it may be too late for them to help. In the case outlined above, our framework would allow the robotic agent to help the human by handing the dishes, regardless of what these dishes are for."
Essentially, instead of predicting a single goal that a human user is trying to tackle, the framework created by the researchers allows an agent to predict a series of goals. This in turn allows a robot or AI assistant to help in ways that are consistent with these goals, without waiting too long before stepping in.
"Common home assistants such as Alexa will only help when asked to," Tianmin Shu, another researcher who carried out the study, told Tech Xplore. "However, humans can help each other in more sophisticated ways. For instance, when you see your partners coming home from the grocery store carrying heavy bags, you might directly help them with these bags. If you wait until your partner asks you to help, then your partner would probably not be happy."
About two decades ago, researchers at the Max Planck Institute for Evolutionary Anthropology showed that the innate tendency of humans to help others in need develops early. In a series of experiments, children as young as 18-months-old could accurately infer the simple intents of others and move to help them achieve their goals.
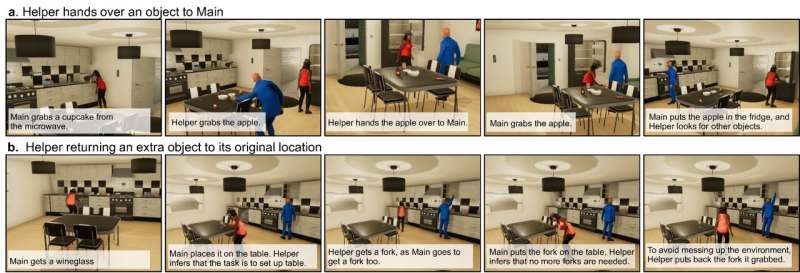
Using their framework, Puig, Shu and their colleagues wanted to equip home assistants with these same "helping abilities," allowing them to automatically infer what humans are trying to do simply by observing them and then act in appropriate ways. This way, humans would no longer need to constantly give instructions to robots and could simply focus on the task at hand.
"NOPA is a method to concurrently infer human goals and assist them in achieving those," Puig and Shu explained. "To infer the goals, we first use a neural network that proposes multiple goals based on what the human has done. We then evaluate these goals using a type of reasoning method called inverse planning. The idea is that for each goal, we can imagine what the rational actions taken by the human to achieve that goal would be; and if the imagined actions are inconsistent with observed actions, we reject that goal proposal."
Essentially, the NOPA framework constantly maintains a set of possible goals that a human might be trying to tackle, constantly updating this set as new human actions are observed. At different points in time, a helping planner then searches for a common subgoal that would be a step forward in solving all the current set of possible goals. Finally, it searches for specific actions that would help to tackle this subgoal.
"For example, the goals could be putting apples inside the fridge, or putting apples on a table," Puig and Shu said. "Instead of randomly guessing a target location and putting apples there, our AI assistant would pick up the apples and deliver them to the human. In this way, we can avoid messing up the environment by helping with the wrong goal, while still saving time and energy for the human."
So far, Puig, Shu and their colleagues evaluated their framework in a simulated environment. While they expected that it would allow agents to assist human users even when their goals were unclear, they had not anticipated some of the interesting behaviors they observed in simulations.
"First, we found that agents were able to correct their behaviors to minimize disruption in the house," Puig explained. "For instance, if they picked an object and later found that such object was not related to the task, they would put the object back in the original place to keep the house tidy. Second, when uncertain about a goal, agents would pick actions that were generally helpful, regardless of the human goal, such as handing a plate to the human instead of committing to bringing it to a table or to a storage cabinet."
In simulations, the framework created by Puig, Shu and their colleagues achieved very promising results. Even if the team initially tuned helper agents to assist models representing human users (to save the time and costs of real-world testing) the agents were found to achieve similar performances when interacting with real humans.
In the future, the NOPA framework could help to enhance the capabilities of both existing and newly developed home assistants. In addition, it could potentially inspire the creation of similar methods to create more intuitive and socially attuned AI.
"So far, we have only evaluated the method in embodied simulations," Shu added. "We would now like to apply the method to real robots in real homes. In addition, we would like to incorporate verbal communication into the framework, so that the AI assistant can better help humans."
More information: Xavier Puig et al, NOPA: Neurally-guided Online Probabilistic Assistance for Building Socially Intelligent Home Assistants, arXiv (2023). DOI: 10.48550/arxiv.2301.05223
Felix Warneken et al, Altruistic Helping in Human Infants and Young Chimpanzees, Science (2006). DOI: 10.1126/science.1121448
© 2023 Science X Network