This article has been reviewed according to Science X's editorial process and policies. Editors have highlighted the following attributes while ensuring the content's credibility:
fact-checked
preprint
trusted source
proofread
Learning to forget—a weapon in the arsenal against harmful AI
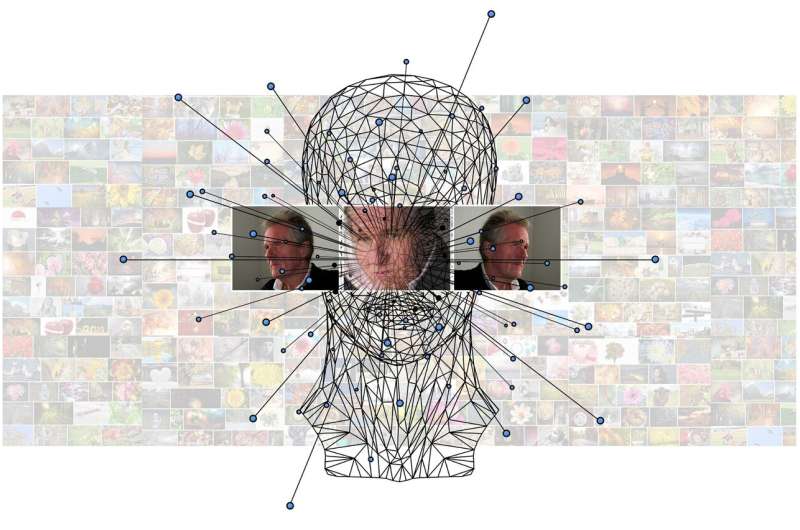
With the AI summit well underway, researchers are keen to raise the very real problem associated with the technology—teaching it how to forget.
Society is now abuzz with modern AI and its exceptional capabilities; we are constantly reminded its potential benefits, across so many areas, permeating practically all facets of our lives—but also its dangers.
In an emerging field of research, scientists are highlighting an important weapon in our arsenal towards mitigating the risks of AI—"machine unlearning." They are helping to figure out new ways of making AI models known as deep neural networks (DNNs) forget data which poses a risk to society.
The problem is re-training AI programs to "forget" data is a very expensive and an arduous task. Modern DNNs such as those based on "Large Language Models" (like ChatGPT, Bard, etc.) require massive resources to be trained—and take weeks or months to do so. They also require tens of Gigawatt-hours of energy for every training program, some research estimating as much energy as to power thousands on households for one year.
Machine Unlearning is a burgeoning field of research that could remove troublesome data from DNNs quickly, cheaply and using less resources. The goal is to do so while continuing to ensure high accuracy. Computer Science experts at the University of Warwick, in collaboration with Google DeepMind, are at the forefront of this research.
Professor Peter Triantafillou, Department of Computer Science, University of Warwick, recently co-authored a publication "Towards Unbounded Machine Unlearning," which appears on the pre-print server arXiv. He said, "DNNs are extremely complex structures, comprised of up to trillions of parameters. Often, we lack a solid understanding of exactly how and why they achieve their goals. Given their complexity, and the complexity and size of the datasets they are trained on, DNNs may be harmful to society.
"DNNs may be harmful, for example, by being trained on data with biases—thus propagating negative stereotypes. The data might reflect existing prejudices, stereotypes and faulty societal assumptions—such as a bias that doctors are male, nurses female—or even racial prejudices.
"DNNs might also contain data with 'erroneous annotations'—for example, the incorrect labeling of items, such as labeling an image as being a deep fake or not.
"Alarmingly, DNNs may be trained on data which violates the privacy of individuals. This poses a huge challenge to mega-tech companies, with significant legislation in place (for example GDPR) which aims to safeguard the right to be forgotten—that is the right of any individual to request that their data be deleted from any dataset and AI program.
"Our recent research has derived a new 'machine unlearning' algorithm that ensures DNNs can forget dodgy data, without compromising overall AI performance. The algorithm can be introduced to the DNN, causing it to specifically forget the data we need it to, without having to re-train it entirely from scratch again. It's the only work that differentiated the needs, requirements, and metrics for success among the three different types of data needed to be forgotten: biases, erroneous annotations and issues of privacy.
"Machine unlearning is an exciting field of research that can be an important tool towards mitigating the risks of AI."
More information: Meghdad Kurmanji et al, Towards Unbounded Machine Unlearning, arXiv (2023). DOI: 10.48550/arxiv.2302.09880