This article has been reviewed according to Science X's editorial process and policies. Editors have highlighted the following attributes while ensuring the content's credibility:
fact-checked
preprint
trusted source
proofread
Researchers create framework for large-scale geospatial exploration
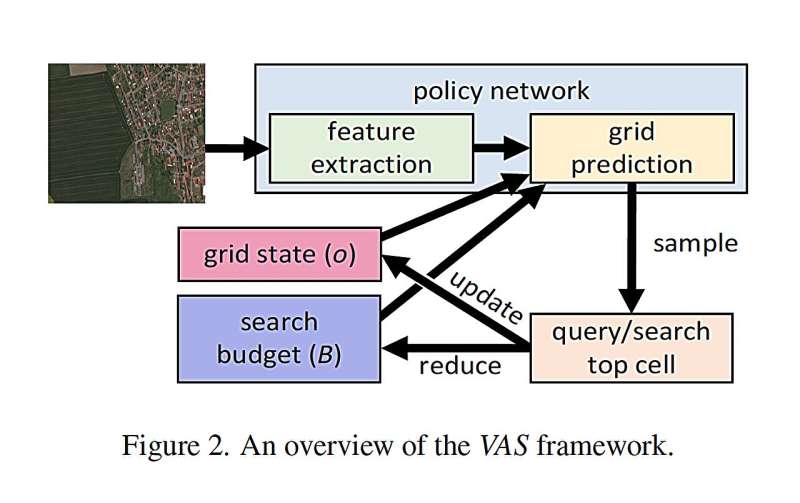
When combating complex problems like illegal poaching and human trafficking, efficient yet broad geospatial search tools can provide critical assistance in finding and stopping the activity. A visual active search (VAS) framework for geospatial exploration developed by researchers in the McKelvey School of Engineering at Washington University in St. Louis uses a novel visual reasoning model and aerial imagery to learn how to search for objects more effectively.
The team led by Yevgeniy Vorobeychik and Nathan Jacobs, professors of computer science & engineering, aims to shift computer vision—a field typically concerned with how computers learn from visual information—toward real-world applications and impact. Their cutting-edge framework combines computer vision with adaptive learning to improve search techniques by using previous searches to inform future searches.
"This work is about how to guide physical search processes when you're constrained in the number of times you can actually search locally," Jacobs said. "For example, if you're only allowed to open five boxes, which do you open first? Then, depending on what you found, where do you search next?"
The team's approach to VAS builds on prior work by collaborator Roman Garnett, associate professor of computer science & engineering at McKelvey Engineering. It marries active search, an area in which Garnett did pioneering research with visual reasoning and relies on teamwork between humans and artificial intelligence (AI). Humans perform local searches, and AI integrates aerial geospatial images and observations from people on the ground to guide subsequent searches.
The novel VAS framework comprises three key components: an image of the entire search area, subdivided into regions; a local search function, determining if a specific object is present in a given region; and a fixed search budget, limiting the number of times the local search function can be executed. The goal is to maximize the detection of objects within the allocated search budget, and indeed, the team found that their approach outperforms all baselines.
First author Anindya Sarkar, a doctoral student in Vorobeychik's lab, presented the findings on Jan. 6 at the Winter Conference on Applications of Computer Vision in Waikoloa, Hawaii. The related research is also available on the arXiv preprint server.
"Our approach uses spatial correlation between regions to scale up and adapt active search to be able to cover large areas," Sarkar said.
"The interactive nature of the framework—learning from prior searches—had been suggested, but updating the underlying model was very costly and ultimately not scalable for a large visual space. Scaling up with lots of image data that's the big contribution of our new VAS method. To do that, we've shifted the underlying fundamentals compared to previous techniques."
Looking ahead, the team anticipates exploring ways to expand their framework for use in various applications, including specializing the model for different domains ranging from wildlife conservation to search and rescue operations to environmental monitoring. They recently presented a highly adaptable version of their search framework that can produce a maximally efficient search even when the object sought varies drastically from the objects the model is trained on.
"The world looks different in different places, and people will want to search for different things," Jacobs said. "Our framework needs to be able to adapt to both of those considerations to be effective as a basis for a search method. We especially want the tool to be able to learn and adapt on the fly since we won't always know what we're looking for or at in advance."
More information: Anindya Sarkar et al, A Visual Active Search Framework for Geospatial Exploration, arXiv (2022). DOI: 10.48550/arxiv.2211.15788