This article has been reviewed according to Science X's editorial process and policies. Editors have highlighted the following attributes while ensuring the content's credibility:
fact-checked
preprint
proofread
Enhancing defect detection performance in smart factories
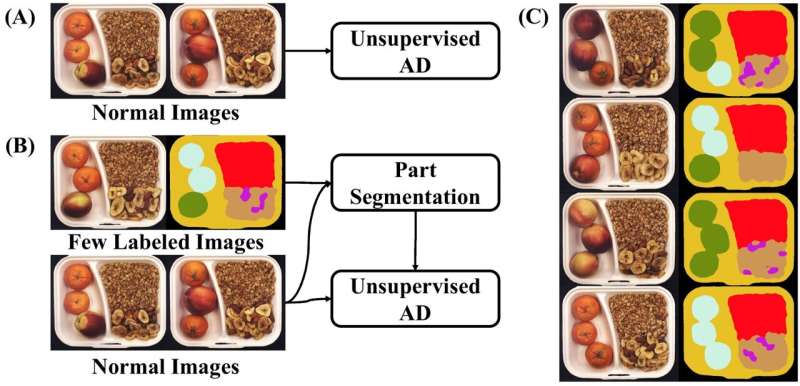
Prof. Sang Hyun Park's research team in the Department of Robotics and Mechatronics Engineering at DGIST has developed a logical anomaly detection technology in collaboration with a team from Stanford University. This technology is expected to significantly improve defect detection performance in smart factories by leveraging AI to identify logical anomalies in industrial images accurately.
Logical anomalies are data that violate basic logical constraints, such as the number, arrangement, or composition of components within an image. Unlike structural anomalies, which can be relatively easily detected by examining partial images, the detection of logical anomalies requires the ability to distinguish among various components throughout the entire image. Previous AI models have recorded poor AUROC scores not exceeding an average of 90% in logical anomaly detection.
To overcome this performance limitation, Prof. Sang Hyun Park's research team has proposed a model that first learns to segment each component accurately and then uses that information to perform anomaly detection.
Typically, training a segmentation model requires pixel-level labeling, which creates a significant labor issue. To address this, the research team has proposed a few-shot segmentation technique utilizing a small number of ground truths.
The images used for model training were combined in the same manner, meaning each image is different, but the number of components or pixels is similar. The segmentation model was effectively trained by minimizing the objective function using histograms. Consequently, the proposed technique demonstrated superior accuracy compared to existing few-shot segmentation techniques.
Furthermore, the research proposes a model that utilizes image segmentation information to perform logical and structural anomaly detection simultaneously. It uses a total of three memory banks to calculate anomaly detection scores through comparison with test images effectively.
The research team applied this technology to the MVTec LOCO AD dataset, which is among the most challenging logical anomaly detection datasets currently available. Whereas the existing techniques have each recorded performance below an average of 90% in logical anomaly detection, the proposed technique achieved an average performance of 98%.
Prof. Sang Hyun Park of the Department of Robotics and Mechatronics Engineering at DGIST said, "This research has dramatically improved performance in logical anomaly detection. This technology could significantly reduce the costs associated with defect detection in smart factories."
The findings are published on the arXiv preprint server.
More information: Soopil Kim et al, Few Shot Part Segmentation Reveals Compositional Logic for Industrial Anomaly Detection, arXiv (2023). DOI: 10.48550/arxiv.2312.13783