March 18, 2024 feature
This article has been reviewed according to Science X's editorial process and policies. Editors have highlighted the following attributes while ensuring the content's credibility:
fact-checked
preprint
trusted source
proofread
A new framework to collect training data and teach robots new manipulation policies
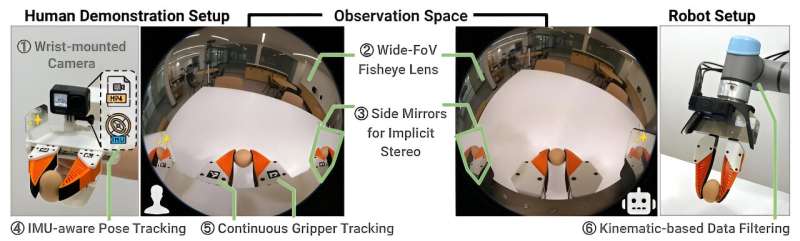
In recent years, roboticists and computer scientists have been trying to develop increasingly efficient methods to teach robots new skills. Many of the methods developed so far, however, require a large amount of training data, such as annotated human demonstrations of how to perform a task.
Researchers at Stanford University, Columbia University and Toyota Research Institute recently developed Universal Manipulation Interface (UMI), a framework to collect training data and transfer skills from human demonstrations in the wild to policies deployable on robots.
This framework, introduced in a paper posted to the preprint server arXiv, could contribute to the advancement of robotic systems, by speeding up and facilitating their training on new object manipulation tasks.
"In the last year, the robotics community saw huge advancement in robotic capability and task complexity, driven by wave of imitation learning algorithms including our prior work 'Diffusion Policy,'" Cheng Chi, co-author of the paper, told Tech Xplore.
"These algorithms take in human teleoperation datasets and produces an end-to-end deep neural network that drives robot actions directly from pixels. These methods are so powerful that we felt with sufficiently large and diverse demonstration datasets, there is no obvious ceiling on their capabilities.
"However, unlike other fields such as natural language processing (NLP) or computer vision (CV), there isn't widely available robotic data on the Internet, thus we have to collect data ourselves."
Compiling large datasets containing a wide range of demonstration data via teleoperation (i.e., the remote operation of physical robots) can be both expensive and time-consuming. Moreover, the logistics required to transport robots complicate the collection of varied data.
Chi and his colleagues set out to tackle these reported challenges of robot training in a scalable and efficient way. The key objective of their recent study was to develop a scalable method to collect real-world robotics training data in a wide range of environments.
"Back in 2020, our lab published a work called 'Grasping in the wild' that pioneered the idea of using a hand-held gripper device, combined with wrist-mounted camera, to collect data in the wild," Chi explained. "However, limited by the learning algorithms at the time as well as some hardware design flaws, the system is limited to simple tasks like object grasping."
Building on their previous works, Chi and his colleagues designed a new system to collect data and train robots. This system, dubbed UMI, includes a hand-held robotic gripper and a deep learning framework that combines the advantageous features of recently developed imitation learning algorithms, such as "Diffusion Policy."
"UMI is a data collection and policy learning framework that allows direct skill transfer from in-the-wild human demonstrations to deployable robot policies," Chi explained. "It consists of two components. The first is a physical interface (i.e., the 3D printed grippers mounted with GoPros) to capture all the information necessary for policy learning while remaining highly intuitive, cost-effective, portable and reliable. The second is a policy interface (i.e., API) that defines a standard way to learn from the data that enables cross-hardware transfer (i.e., deploying to multiple real-world robots)."
The framework developed by Chi and his collaborators has numerous advantages over other methods to collect data and train robotic manipulators. First, the UMI grippers they developed were much more intuitive than previously introduced teleoperation approaches.
"A data collector can demonstrate much harder tasks much faster compared to teleportation," Chi said, "As a result, the learned policy becomes more effective."
The second advantage of UMI is that it enables the collection of large and diverse datasets that allow robots to generalize well across unseen environments and object manipulation tasks. Collecting this data using UMI is also far cheaper and more feasible than compiling annotated training datasets using conventional methods.
"UMI also enables cross-hardware generalization," Chi said. "Any research lab can retrofit their industrial robot arms with UMI-compatible grippers and cameras, and directly deploy the policies we trained, or take advantage of the data we collected for pre-training. In comparison, most of the dataset that currently exists are specific to a robot embodiment and often to a specific lab environment. As a result, UMI could enable large-scale robotic data sharing across academia, similarly to datasets used in NLP and CV community."
In initial experiments, the UMI approach yielded very promising results. It was found to enable highly intuitive end-to-end imitation learning, training robots on various complex manipulation tasks with limited engineering efforts on the part of researchers, including dishwashing and folding clothes.
"Our experiments also showed that, with diverse data, end-to-end imitation learning can generalize to in-the-wild, unseen environments and unseen objects," Chi said. "In contrast, the standard for evaluating these end-to-end imitation learning methods previously has been using the same environment for both training and testing. Collectively, the evidence we collected suggests that with sufficiently large and diverse robotics dataset, general-purpose robots such as home robots might become feasible, even without a paradigm change on learning algorithms."
The new framework introduced by Chi and his collaborators could soon be used to collect other training datasets and tested on a wider range of complex manipulation tasks. The design of the UMI gripper and its underlying software are open-source and can be accessed by other teams on GitHub.
"We now wish to further expand the capabilities and observation modalities of UMI, by improving the hardware and adapting them to a broader range of robots," Chi added. "We also plan to collect even more data and use those data to further improve learning algorithms."
More information: Cheng Chi et al, Universal Manipulation Interface: In-The-Wild Robot Teaching Without In-The-Wild Robots, arXiv (2024). DOI: 10.48550/arxiv.2402.10329
© 2024 Science X Network