A deep-learned e-skin decodes complex human motion
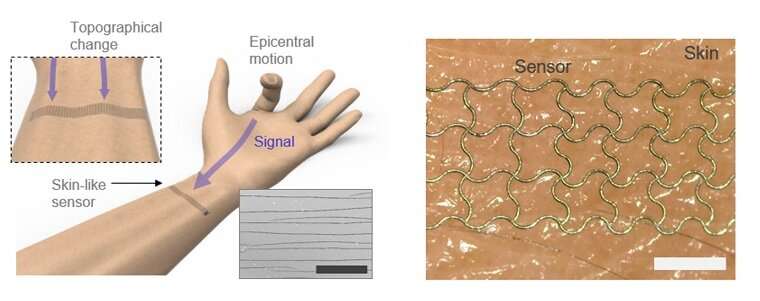
A deep-learning powered single-strained electronic skin sensor can capture human motion from a distance. The single strain sensor placed on the wrist decodes complex five-finger motions in real time with a virtual 3-D hand that mirrors the original motions. The deep neural network boosted by rapid situation learning (RSL) ensures stable operation regardless of its position on the surface of the skin.
Conventional approaches require many sensor networks that cover the entire curvilinear surfaces of the target area. Unlike conventional wafer-based fabrication, this laser fabrication provides a new sensing paradigm for motion tracking.
The research team, led by Professor Sungho Jo from the School of Computing, collaborated with Professor Seunghwan Ko from Seoul National University to design this new measuring system that extracts signals corresponding to multiple finger motions by generating cracks in metal nanoparticle films using laser technology. The sensor patch was then attached to a user's wrist to detect the movement of the fingers.
The concept of this research started from the idea that pinpointing a single area would be more efficient for identifying movements than affixing sensors to every joint and muscle. To make this targeting strategy work, it needs to accurately capture the signals from different areas at the point where they all converge, and then decoupling the information entangled in the converged signals. To maximize users' usability and mobility, the research team used a single-channeled sensor to generate the signals corresponding to complex hand motions.
The rapid situation learning (RSL) system collects data from arbitrary parts on the wrist and automatically trains the model in a real-time demonstration with a virtual 3-D hand that mirrors the original motions. To enhance the sensitivity of the sensor, researchers used laser-induced nanoscale cracking.
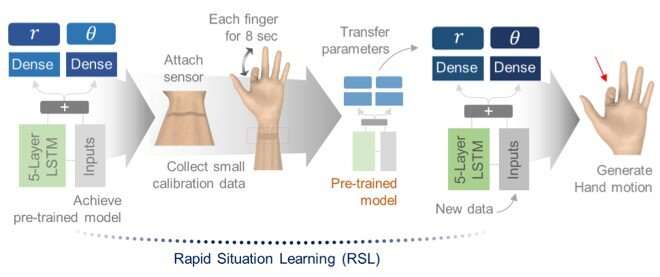
This sensory system can track the motion of the entire body with a small sensory network and facilitate the indirect remote measurement of human motions, which is applicable for wearable VR/AR systems.
The research team said they focused on two tasks while developing the sensor. First, they analyzed the sensor signal patterns into a latent space encapsulating temporal sensor behavior and then they mapped the latent vectors to finger motion metric spaces.
Professor Jo said, "Our system is expandable to other body parts. We already confirmed that the sensor is also capable of extracting gait motions from a pelvis. This technology is expected to provide a turning point in health-monitoring, motion tracking, and soft robotics."
More information: Kim, K. K., et al. (2020) A deep-learned skin sensor decoding the epicentral human motions. Nature Communications. 11. 2149. doi.org/10.1038/s41467-020-16040-y29