Accurate and efficient 3-D motion tracking using deep learning
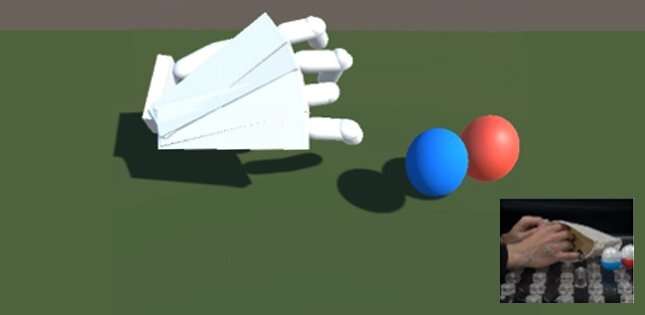
A new sensing method has made tracking movement easier and more efficient. A research group from Tohoku University has captured dexterous 3-D motion data from a flexible magnetic flux sensor array, using deep learning and a structure-aware temporal bilateral filter.
Dexterous 3-D motion data can be used for multiple purposes: biologists can use the data to record detailed movements of small animals in their living environments, scientists can track the flow of fluids, and researchers can track finger movements and objects being manipulated by users in virtual reality.
Currently, optical cameras are the most prominent method of tracking movements. Yet optical cameras struggle with accuracy and reliability. If a small animal burrows away or if fingers or objects obscure the view, the camera will fail to detect the motion.
Magnetic tracking technology is also used for dexterous motion. However, even state-of-the-art magnetic systems face limitations. The classic tracking method creates bias and magnetic sources have a dead-angle problem or bulky markers.
The research team invented their new method by applying a deep neural network and a novel structure-aware temporal bilateral filter on a new magnetic tracking principle. First, the neural networks learn the regression from the simulation flux values to the LC coils 3-D configuration at any location and orientation.
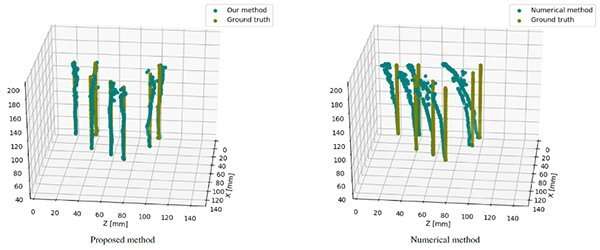
The new filter further compensates the data to reconstruct smooth and accurate motion. Markers do not require batteries, so observation time can be maximized.
As a result, the new integrated system can track multiple LC coils at 100Hz speed at millimetre level accuracy. Tracking loss due to dead-angle can be reconstructed because of the system's self-learning.
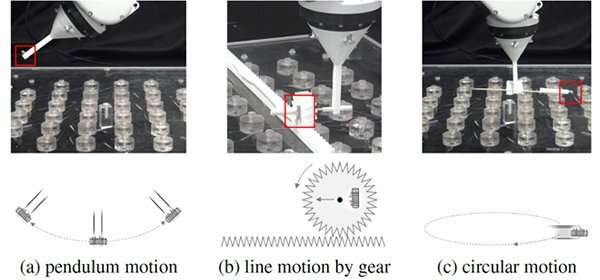
"The application of our research is widespread. Hand motions can be tracked to make creating smooth animations easier, markers can be put into fluids to track its flow, and tracking can be placed on small animals," added Kitamura.
More information: Jiawei Huang et al. Reconstruction of Dexterous 3D Motion Data from a Flexible Magnetic Sensor with Deep Learning and Structure-Aware Filtering, IEEE Transactions on Visualization and Computer Graphics (2020). DOI: 10.1109/TVCG.2020.3031632