December 14, 2023 feature
This article has been reviewed according to Science X's editorial process and policies. Editors have highlighted the following attributes while ensuring the content's credibility:
fact-checked
preprint
trusted source
proofread
Gathering more effective human demonstrations to teach robots new skills
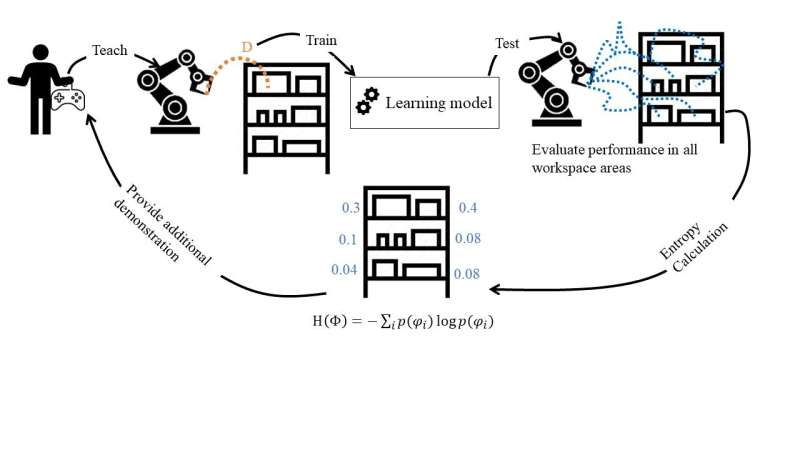
To effectively assist humans in real-world settings, robots should be able to learn new skills and adapt their actions based on what users require them to do at different times. One way to achieve this would be to design computational approaches that allow robots to learn from human demonstrations, for instance observing videos of a person washing dishes and learning to repeat the same sequence of actions.
Researchers at University of British Columbia, Carnegie Mellon University, Monash University and University of Victoria recently set out to gather more reliable data to train robots via demonstrations. Their paper, posted to the arXiv preprint server, shows that the data they gathered can significantly improve the efficiency with which robots learn from the demonstrations of human users.
"Robots can build cars, gather the items for shopping orders in busy warehouses, vacuum floors, and keep the hospital shelves stocked with supplies," Maram Sakr, one of the researchers who carried out the study, told Tech Xplore. "Traditional robot programming systems require an expert programmer to develop a robot controller that is capable of such tasks while responding to any situation the robot may face."
Conventional approaches for training robots to complete specific tasks require the skills of computer scientists. Often, to work well these approaches require tasks to be broken down into tens or hundreds of smaller sub-tasks, subsequently testing the robustness of each of these sub-tasks.
This process is both time consuming and computationally demanding. In addition, if a failure occurs and the learning model stops working properly, it will need to be fixed by highly skilled technicians.
"Learning from demonstrations (LfD) is a promising alternative approach for training robots that allows non-expert human teachers (i.e., domain experts but not robotics experts) to program the robot simply by showing it how to perform the task; no programming is required," Sakr said. "Then, when failures occur, the human teacher only needs to provide more demonstrations, rather than calling for professional help. LfD seeks to endow robots with the ability to learn how to perform a task by generalizing from several observations of a human teacher."
LfD methods build on state-of-the-art machine learning (ML) techniques that achieved remarkable results on various tasks. The effective training of these techniques relies on effective and good quality demonstration data, yet most available datasets contain low-resolution, low quality or insufficient footage.
"Collecting the training dataset in any learning system is critical to a successful learning process," Sakr said. "The training data must be representative of the states that the robot will encounter in the future. Thus, this paper aims to guide users to provide an efficient set of demonstrations for the robot to learn from. By 'efficient' we mean the minimum number of demonstrations that are well-distributed over the task space to achieve high generalization capabilities for the robot."
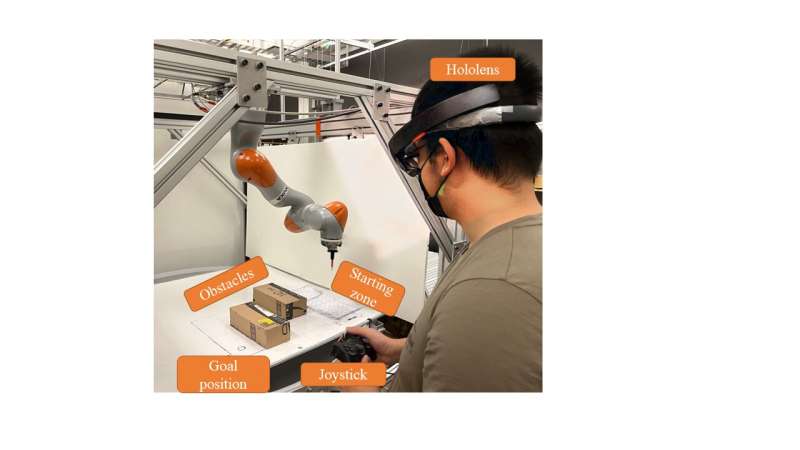
A key limitation of previously proposed LfD approaches is that they rely on demonstrations performed by computer scientists, rather than by everyday non-expert users. In their paper, Sakr and her colleagues explore the possibility of teaching everyday users to select training data or demonstrations that enhance a robot's learning and allow it to generalize better across different tasks.
"During human teacher training, areas in the task space with the highest uncertainty regarding the robot's ability to perform the task are highlighted," Sakr explained. "Additional demonstrations in these areas could benefit the robot the most in executing the task successfully while using the teacher's effort efficiently (i.e., providing a lower number of demonstrations that achieve wider generalization for the robot). Under this guidance, the human teacher can observe which next demonstration maximizes robot learning, as well as the size and diversity of the demonstrations needed to fully cover the workspace."
Notably, the criteria for selecting effective demonstrations outlined by Sakr and her colleagues can be easily followed by various human users, regardless of their level of expertise and of the specific algorithm powering a robot. If a user provides low-quality or ineffective demonstrations, the proposed guidance system will highlight the need for a greater number of demonstrations to enhance the robot's learning.
The researchers assessed the effectiveness of their approach in a simple experiment, where 24 novice robot users were trained to produce effective demonstrations using an augmented reality (AR)-based guidance system based on their criteria. After these non-expert users completed their training, the team assessed their ability to create effective demonstrations on new trials that focused on new tasks, without providing any guidance.
"We demonstrated that a brief session of interactive training and guidance significantly enhanced lay users' teaching skills, leading to improved robot learning and generalization efficiency," Sakr said. "Notably, this online learning occurred through demonstrations from a teacher without prior knowledge of robotics or machine learning algorithms. The proposed training framework allows users to grasp the required demonstrations for efficient robot learning without delving into the intricacies of the learning process."
The results gathered by Sakr and their colleagues suggest that teaching non-expert users to create effective demonstrations could significantly reduce the cost of training robots via imitation learning, while also increasing the efficiency with which they learn. The team found that demonstrations created by their trained participants improved the efficiency at which the robots learned by up to 198% compared to demonstrations created by non-trained users and by 210% when compared to learning approaches based on trial and error.
"Our research aims to democratize access to robotics across all domains," Sakr said. "Integrating intuitive and interactive training into the LfD pipeline has the potential to greatly expand the use of robots in various fields. This approach can enhance human-robot interaction by reducing the time needed to train a robot for a new task. Moreover, it facilitates skill transfer for domain experts who lack programming knowledge."
In the future, the criteria and AR-based guidance system employed by this team of researchers could help to better teach robots new skills via non-expert demonstrations. In addition, the recent work by Sakr and her colleagues could inspire other teams to develop similar approaches to create effective task demonstrations, ultimately facilitating the deployment of robots in real-world environments and enhancing their ability to learn from observing humans
"The significant improvement in the efficiency by only guiding users to well distribute the demonstrations suggests that guiding users to provide high-quality demonstrations along with their good distribution could further boost learning efficiency," Sakr added. "Testing the proposed approach in real-world facilities with users under uncontrolled conditions would be intriguing. In such scenarios, users could decide the duration of guidance or use it consistently to ensure they offer the most beneficial demonstrations to the robot.
"Finally, exploring the application of the entropy-based guidance system in different domains and in combination with various learning algorithms presents an opportunity to assess its generalization capabilities further."
More information: Maram Sakr et al, How Can Everyday Users Efficiently Teach Robots by Demonstrations?, arXiv (2023). DOI: 10.48550/arxiv.2310.13083
© 2023 Science X Network