April 5, 2024 feature
This article has been reviewed according to Science X's editorial process and policies. Editors have highlighted the following attributes while ensuring the content's credibility:
fact-checked
preprint
trusted source
proofread
A framework to improve air-ground robot navigation in complex occlusion-prone environments
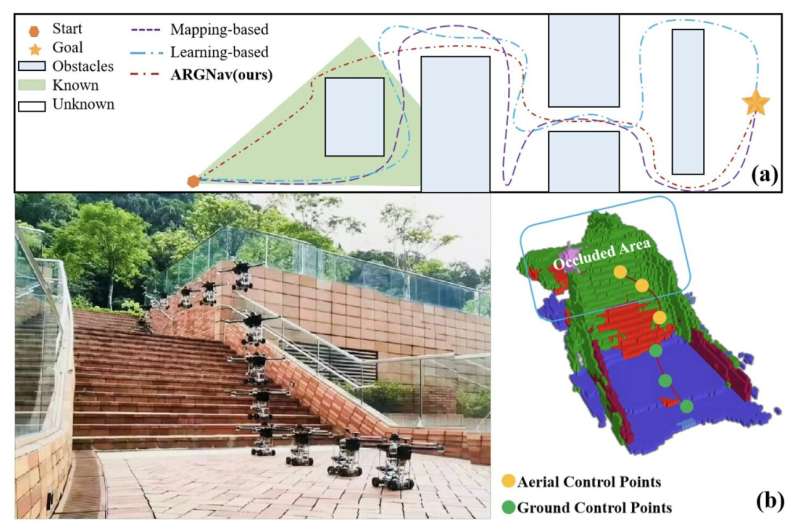
Robotic systems have so far been primarily deployed in warehouses, airports, malls, offices, and other indoor environments, where they assist humans with basic manual tasks or answer simple queries. In the future, however, they could also be deployed in unknown and unmapped environments, where obstacles can easily occlude their sensors, increasing the risk of collisions.
Air-ground robots could be particularly effective for navigating outdoor environments and tackling complex tasks. By moving both on the ground and in the air, these robots could help humans search for survivors after natural disasters, deliver packages to remote locations, monitor natural environments, and complete other missions in complex outdoor settings.
Researchers at University of Hong Kong have recently developed AGRNav, a new framework designed to enhance the autonomous navigation of air-ground robots in occlusion-prone environments. This framework, introduced in a paper published on the arXiv preprint server, was found to achieve promising results both in simulations and real-world experiments.
"The exceptional mobility and long endurance of air-ground robots are raising interest in their usage to navigate complex environments (e.g., forests and large buildings)," Junming Wang, Zekai Sun, and their colleagues wrote in their paper. "However, such environments often contain occluded and unknown regions, and without accurate prediction of unobserved obstacles, the movement of the air-ground robot often suffers a suboptimal trajectory under existing mapping-based and learning-based navigation methods."
The primary objective of the recent study by this team was to develop a computational approach to enhance the navigation of air-ground robots in settings where parts of their surroundings are easily occluded by objects, vehicles, animals, and other obstacles. AGRNav, the framework they developed, has two main components: a lightweight semantic scene completion network (SCONet) and a hierarchical path planner.
The SCONet component predicts the distribution of obstacles in an environment and their semantic features, using a deep learning approach that only performs a few calculations. The hierarchical path planner, on the other hand, uses the predictions made by SCONet to plan optimal, energy-efficient aerial and ground paths for a robot reach a given location.
"We present AGRNav, a novel framework designed to search for safe and energy-saving air-ground hybrid paths," the researchers wrote. "AGRNav contains a lightweight semantic scene completion network (SCONet) with self-attention to enable accurate obstacle predictions by capturing contextual information and occlusion area features. The framework subsequently employs a query-based method for low-latency updates of prediction results to the grid map. Finally, based on the updated map, the hierarchical path planner efficiently searches for energy-saving paths for navigation."
The researchers evaluated their framework in both simulations and real-world environments, applying it to a customized air-ground robot they developed. They found that it outperformed all the baseline and state-of-the-art robot navigation frameworks to which it was compared, identifying optimal and energy-efficient paths for the robot.
AGRNav's underlying code is open-source and can be accessed by developers worldwide on GitHub. In the future, it could be deployed and tested on other air-ground robotic platforms, potentially contributing to their effective deployment in real-word environments.
More information: Junming Wang et al, AGRNav: Efficient and Energy-Saving Autonomous Navigation for Air-Ground Robots in Occlusion-Prone Environments, arXiv (2024). DOI: 10.48550/arxiv.2403.11607
© 2024 Science X Network